The scaling properties of multi-unit soft sensors
Industrial processes often consist of replicated process units, such as pumps, compressors, and tanks. This is because profit margins depend on production at scale, which often requires parallel production lines. Our most recent research explores the advantages of utilizing data from multiple, similar process units when developing soft sensors. This is the first of two blog posts, where we present the highlights of this research and demonstrate its application to data-driven virtual flow metering in oil and gas.
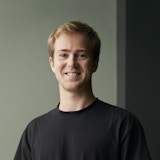
- Author
- Jens Nikolai Alfsen
- Publish date
- · 3 min read
A soft sensor, also known as a virtual sensor, is a system used in industrial process environments to infer the value of a hard-to-measure variable. The sensor is essentially a mathematical model that takes more readily available measurements as input. The main assumptions for the input measurements are that they contain relevant information about the hard-to-measure variable and that they are more frequently available.
The motivation for developing an on-line soft sensor is typically to monitor some key performance indicator. The process can then be optimized in real time - based on the inferred values. There are two established approaches to developing a soft sensor; model-based, in which the underlying process is modeled from first-principle physics, and data-driven which has gained significant traction in recent years. Due to the typical complexity of the process and expensive maintenance of a model-based soft sensor, a data-driven approach is proposed. The main challenge, though, is that a soft sensor is most valuable in information-poor environments, where one would expect data-driven methods to perform poorly. To achieve a good predictive performance in such environments, it is imperative to be data efficient.
«The main challenge is that a soft sensor is most valuable in information-poor environments, where one would expect data-driven methods to perform poorly. To achieve a good predictive performance in such environments, it is imperative to be data efficient.»
A data-driven soft sensor is a type of soft sensor that primarily relies on data to make estimates or predictions about a process or a system. Unlike model-based soft sensors, which are built upon theoretical models and understanding of the process mechanics, data-driven soft sensors utilize statistical and machine learning methods to learn from historical data.
The proposed solution is inspired by transfer learning, the technique of taking knowledge acquired by solving a source task and utilizing it to improve generalization on a target task. Applied to the soft sensor challenge, the unidirectional transfer of knowledge is generalized to be multidirectional. Multiple soft sensors, or units, are learned simultaneously and knowledge transfer is enabled through parameter sharing.
«The soft sensor performance increases with the number of units. The convergence rate matches a theoretical estimate, and can be used to indicate when a good base model is found.»

The hypothesis of increasing soft sensor performance is tested in an empirical study on the problem of Virtual Flow Metering. In the study, the average soft sensor performance is, in fact, shown to increase as expected with the number of soft sensors (M). The figure shows the empirical result against theoretical expected learning rate as a function of the number of units (M) in the dataset. Notice the diminishing returns after M ≃ 40. Also worth noticing is that for M=80, the test error is about half that of single-unit soft sensors (M=1).
In an upcoming article, we will see how this insight can be used to develop data-driven soft sensors which work using extremely little data. Read the full paper at Multi-unit soft sensing permits few-shot learning.
References
- Naiju Zhai et al. “Soft Sensor Model for Billet Temperature in Multiple Heating Furnaces Based on Transfer Learning”. en. In: IEEE Transactions on Instrumentation and Measurement 72 (2023), pp. 1–13. issn: 0018-9456, 1557-9662. doi: 10 . 1109 / TIM . 2023 . 3267520. (Visited on 08/18/2023).
- Yuxin Huang et al. “Modeling Task Relationships in Multivariate Soft Sensor With Balanced Mixture-of-Experts”. en. In: IEEE Transactions on Industrial Informatics 19.5 (May 2023), pp. 6556–6564. issn: 1551-3203, 1941-0050. doi: 10.1109/TII.2022.3202909. (Visited on 08/18/2023).
- Yu Zhang and Qiang Yang. “A Survey on Multi-Task Learning”. In: IEEE Transactions on Knowledge and Data Engineering 4347.c (2021), pp. 1–20. doi: 10.1109/TKDE.2021.3070203.
- Bjarne Grimstad et al. "Multi-unit soft sensing permits few-shot learning"